The 6th Carbon Budget, released in December 2020, sets out national decarbonisation pathways to 2050 across all sectors. Following these pathways, we can expect an installation rate of about 415,000 heat pumps per year by 2025, rising to over 1 million installations per year by 2030. Electrification of space heating is gathering pace, and with it, intelligent energy management is moving from being beneficial to being necessary to ensure the resilience of the power grid.
The Challenge of Scale
At this scale, the roll-out of bespoke control strategies in buildings is an enormous challenge. As noted previously, the need for modelling and optimisation expertise is a significant roadblock. To overcome it, we must lighten the control design burden of the modeller and lean instead on data.
Data-driven control
The increasing role of data across the technology spectrum is familiar to us all. In the built environment, it could be transformational, but we need suitable tools with which we can distil data into knowledge, and knowledge into actionable decisions. In short, we want data-driven control.
The realisation of such technology comes with pitfalls, however. Energy system usage tends to be characterised by repetitive schedules and static operating points. Historical data often don’t contain the information content necessary to inform many of the complex modelling paradigms common in the wider field of data science. On the other hand, successful control-oriented methods have largely relied on the field of System Identification, which still requires a level of designer interaction.
The Behavioural Systems Theory Approach
In the mid-2000s a new perspective on system modelling was proposed by Jan Willems called Behavioural Systems Theory. This theory views behaviour as the set of all possible time trajectories, subject to the constraints imposed by the physics of a dynamic system. In the past year, a flurry of activity in the control community has seen the principles of this theory put to use in the development of purely data-driven control methods that remove the system identification step from the design process.
Crucially, these methods rely on a level of data excitation that is quantifiable and often not difficult to achieve for linear systems. In the building energy domain, in which linear heat transfer dominates, this makes them a natural fit.
Applying these methods to heating systems
Our team at Imperial College has been developing these concepts further to better suit the requirement for rapid control implementation in buildings. We have devised an algorithm called Segmented Data-driven Predictive Control suited to longer predictions, which allows us to use day-ahead predictions suited to the building control problem (a paper exploring this in more detail can be accessed here). The algorithm also reduces the required training time (a few hours) while speeding up computation time. Our studies suggest that we can achieve comfort and energy performance comparable to that of system identification-based approaches, without requiring a bespoke design stage.
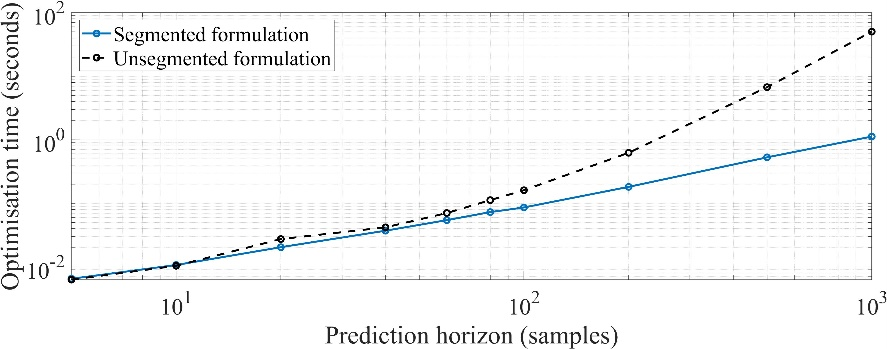
Moving to the real world
These methods can help us to overcome the design challenge we face in the roll-out of intelligent control, but refinements are needed. Such heavy reliance on data leaves us open to problems if the data quality is low. To be robust to noise and any other unexpected anomalies that can occur, we need algorithms that can learn and improve over time. Furthermore, users change, buildings change, and our controllers must adapt accordingly. We use measurements to manage our energy systems. If these measurements can tell us a story, we need the tools to comprehend it.
Dr Edward O’Dwyer (e.odwyer@imperial.ac.uk) is a Research Associate with the Sargent Centre for Process Systems Engineering at Imperial College London. Research on the Active Building Centre Research Programme has been conducted in close collaboration with the Department of Electrical and Electronic Engineering of Imperial College London.