Real life is uncertain, yet, despite everyone’s best efforts, it is impossible to remove the effects of uncertainty from our daily lives. Or is it? Well, if we are talking about the operation of residential buildings, we can. The team at Imperial College London are developing robust control strategies that will help to achieve low emissions goals and optimal comfort, while accounting for uncertainty.
Where is the uncertainty?
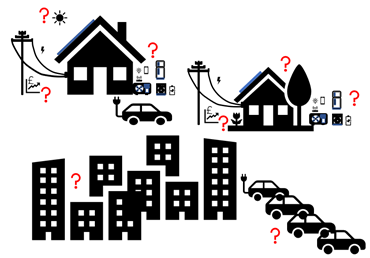
Let us first take a look at what the sources of operational uncertainty in residential buildings are. If you have ever planned an activity based on a weather forecast, you know that these forecasts should be taken with caution. Even more so in the case of long-term forecasts; predicting what the weather will be a year from now can be an educated guess at best (I mean, the temperature in December is supposed to be low, isn’t it?). Thus, one source of uncertainty in the operation of residential buildings is the prediction of the external conditions.
Another source of uncertainty is what we actually know about a building. The number of rooms in a flat, or the number of floors in a block of flats is obvious, I hear you say. But do we know how thick the walls are? Or what the walls are made of? Or how much heat escapes from each flat, or the block as whole, because of leaky windows?
The ABC-RP modelling team are developing new ways to describe the behaviour of residential buildings with greater accuracy, and they are providing insights into the second source of uncertainty which is the mismatch between the models and real buildings.
Finally, as the famous IT saying goes, “the problem exists between chair and computer”. In this case, it is rather that building occupants are unpredictable rather than the building itself. The needs of occupants vary from household to household, from day to day, from season to season. On top of these variables, active buildings interact with the electricity grid to satisfy not only the occupant, but also the grid. Thus, the demand from the occupants and interactions with the grid are the third source of uncertainty that must be considered.
Robust control to the rescue
So how can we deal with these sources of uncertainty and at the same time reduce emissions from residential buildings? We could devise a set of rules, like “if the temperature in a room is below a certain value, increase the heating”, “buy electricity when the price is low, sell it when the price is high”, or “turn the lights off as soon as the user leaves a room”.
Well, the first rule is dependent on individual preferences of the user, the second rule is easier said than done, and the third rule is likely to result in building occupants unhappy about constantly flashing lights. And how to ensure that the environmental goals are achieved using this set of rules? And how do we include uncertain factors like those we discussed earlier?
Fortunately, there exist control strategies that can take care of the environmental goals, as well as take uncertainty into account. The strategy developed by our team at Imperial College London is based on Model Predictive Control (MPC), which has already been shown useful in mitigating energy consumption in residential housing.
With our knowledge of uncertainty, we come up with a set of scenarios and then find a control strategy that provides optimal results in all of them. You can think about these scenarios like answering multiple questions from a precocious toddler: what happens if the temperature in December is low? And what happens if it stays high? And what happens if we lose the thermometer and cannot measure the temperature anymore?
The idea of scenario-based MPC is quite simple and aims to iteratively optimise a buildings performance:
- Devise a control strategy for a given time horizon and predefined scenarios, using our current knowledge about the operating conditions and the building. For example, we propose a control strategy for a week into the future assuming that the temperature will stay in a given range, the prices will be low, and the occupants may go on holiday.
- Apply the control strategy over a shorter time horizon. For example, if our control strategy was devised for a week, we use it only for the first day. At the end of that day, we will know more about the system, as well as which scenario occurred.
- Update our knowledge about the system and the possible scenarios based on the outcome of step 2, and go back to step 1 to devise a new control strategy. In other words, we feed our new knowledge back into the system to improve the control strategy.
The question is how to apply this idea in real life. It would get boring pretty quickly if we had to come up with a new control strategy manually every day, especially if the number of scenarios is large (and it will be, it is like a really keen toddler). Instead, we use mathematics to optimise over feedback in real-time while playing out these scenarios. This approach allows us to continuously update the control strategies without explicitly specifying the rules, and thus reduce the impact of uncertainty.
Preliminary results show that scenario-based MPC leads to improved operation of residential buildings that are subject to uncertainty factors. We are now developing new, open-source solutions that will provide efficient operating strategies for residential buildings regardless of how much uncertainty there is.
Uncertainty? Yes please, but with control!
Marta Zagorowska is a research associate at Imperial College London where she focuses on control and optimisation of systems for buildings as part of the Active Building Research Programme.